Chandan Singh, Chief Product Officer, Thinkdeeply
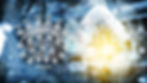
AI and the challenge of model explainability
Artificial intelligence (AI) has become increasingly pervasive and is experiencing widespread adoption in all industries. Faced with increasing competitive pressures and observing the AI success stories of their peers, more and more organizations are adopting AI in various facets of their business. Machine Learning (ML) models, the key component driving the AI systems, are becoming increasingly powerful, displaying superhuman capabilities on most tasks. However, this increased performance has been accompanied by an increase in model complexity, turning the AI systems into a black box whose decisions can be hard to understand by humans. Employing black box models can have severe ramifications, as the decisions made by the systems not only influence the business outcomes but can also impact many lives. From driving cars and preventing crime to product recommendations, making investment decisions, approving loans, and hiring employees, ML models are increasingly being employed to replace human decision-making. So it becomes increasingly important for stakeholders to understand how these algorithms make their decisions to gain the trust and confidence in the use of AI in their operations. As a result, there has been an increased interest in Explainable Artificial Intelligence (XAI), a field concerned with the development of methods that explain and help interpret machine learning models.
What is Explainable AI?
The field of Explainable AI (XAI) is focused on developing tools, frameworks, and methods that help understand how machine learning models make decisions. Its goal is to provide insights into the inner workings of the complex ML models and help understand the logic that goes into the model's decision-making. XAI helps bring transparency to AI, making it possible to open up the black box and reveal the decision-making process in an easily understandable way to humans. The model explanations are typically extra metadata information in the form of some visual or textual guides that offer insight into specific AI decisions or reveal the internal functionality of the model as a whole. The mechanisms of expressing the metadata include text explanations, visual explanations, explanations by example, explanations by simplification, and feature relevance explanations. XAI is a fast-evolving field, and there is already immense literature on explainability mechanisms and techniques. I have provided some references at the end of this article. The focus of this writing is on building the business case for Explainable AI.
Why is model explainability important?
Fairness, trust, and transparency are the three primary concerns driving the need for explainability. AI systems have been found to produce unfair, biased, and unethical decisions in many instances (Robert, Pierce, et al., 2020). For example, AI systems screening applicants have been shown to be biased against hiring women and other minorities, like Amazon's recruitment engine that exhibited biases against female applicants (Amazon scraps secret AI recruiting tool that showed bias against women). Fairness is undermined when managers rely blindly on AI outputs to augment or replace their decision-making without knowing how and why the model made those decisions, how the model was trained, what was the quality of dataset used, or when does and when does the model not work well. By providing insights into the workings of models, XAI promotes Fairness and helps mitigate biases that can be introduced either from input datasets or poor model architecture.
Trust is another important factor as the complexity of the model and the impact of its decisions increase. It is hard to trust the decisions of systems that one cannot observe and understand. For example, how confident would a doctor or the patient feel about following the recommendations of an AI algorithm giving a diagnosis without having clarity on why the algorithm made those recommendations? An AI diagnosis may prove to be more accurate, but a lack of explainability would create a lack of trust and hence the hesitation to use. The explainability of models can help build trust in its outcomes and cement stakeholder's confidence in its use.
Transparency is the third key factor driving the need for explainability. Transparency helps to assess the quality of output predictions, understand the risks associated with the model use, and be informed of scenarios in which the model may not perform well. By gaining an intuitive understanding of a model's behavior, the individuals responsible for the model can identify scenarios where the model is likely to fail and take the appropriate action. It can also help deter adversarial attacks by making business users aware of ways in which model inputs can be manipulated to influence the outputs
Besides improving Fairness, trust, and transparency, explainability can also help in improving the model performance by providing an understanding of its potential weaknesses. Understanding why and how the model works and why it sometimes fails enables the ML engineers to improve and optimize it. For example, understanding the model behavior for different input data distributions could help explain the skewness and biases in the input data that ML engineers can use to make adjustments and generate a more robust and fair model.
The business value of Explainable AI
Explainable AI also has strategic value for business leaders. Explainability can accelerate AI adoption, enable accountability, provide strategic insights, and ensure ethics and compliance. As explainability helps build the trust and confidence of stakeholders in the ML, it increases the adoption of AI systems in the organization providing it a competitive advantage. Explainability gives confidence to organizational leaders to accept the accountability for the AI systems in their business as it provides them a better understanding of the systems' behavior and potential risks. This promotes greater executive buy-in and sponsorship for AI projects. With the support of key stakeholders and executives for AI, the organization will be better positioned to foster innovation, transformation, and developing next-generation capabilities.
Explainable models can also help provide valuable insights into key business metrics such as sales, customer churn, product reputation, employee turnover, etc., which can improve decision-making and strategy planning. For example, many companies employ machine learning models to measure customer sentiment. While understanding customer sentiment is valuable, a model explanation can also provide insights into the drivers of the sentiment like price, customer service, product quality, etc., and their effect on the customer, allowing businesses to take appropriate steps to address the issues. Similarly, sales forecasting models are used by many companies to predict sales and plan inventory. If the forecasting models can also show how the key factors like price, promotion, competition, etc., contribute to sales forecast, that information can be used to boost sales.
Regulatory compliance is forcing some businesses to adopt Explainable AI practices (New AI Regulations Are Coming. Is Your Organization Ready?). Organizations face growing pressure from customers, regulators, and industry consortiums to ensure their AI technologies align with ethical norms and operate within publicly acceptable boundaries. Regulatory priorities include safeguarding vulnerable consumers, ensuring data privacy, promoting ethical behavior, and preventing bias. Models that exhibit unintentional demographic bias are of particular concern. The use of explainable models is one way of checking for bias and decision-making that doesn't violate ethical norms of business and prevent reputation loss. From a data privacy point of view, XAI can help to ensure only permitted data is being used in model training for an agreed purpose and make it possible to delete data if required. It is important to build a moral compass in AI training from the outset and monitor AI behavior thereafter through XAI evaluation.
Explainable AI should be a required element of an organization's AI principles
With explainability being such a critical requirement, it is imperative for explainable AI to be included in every organization's AI principles and be a key consideration in their AI strategy. Explainability cannot be an afterthought and must be planned right from the start and integrated into the entire ML lifecycle. A formal mechanism that aligns a company's AI design and development with its ethical values, principles,
and risk appetite may be necessary. It is important to ensure that business managers understand the risks and the limitations of unexplained models and are able to take accountability for the risks.
References:
Some good journal articles and textbook on Explainability methods and techniques:
Linardatos, P., Papastefanopoulos, V., & Kotsiantis, S. (2021). Explainable ai: A review of machine learning interpretability methods. Entropy, 23(1), 18.
Arrieta, A. B., Díaz-Rodríguez, N., Del Ser, J., Bennetot, A., Tabik, S., Barbado, A., ... & Herrera, F. (2020). Explainable Artificial Intelligence (XAI): Concepts, taxonomies, opportunities and challenges toward responsible AI. Information Fusion, 58, 82-115.
Samek, W., Montavon, G., Vedaldi, A., Hansen, L. K., & Müller, K. R. (Eds.). (2019). Explainable AI: interpreting, explaining and visualizing deep learning (Vol. 11700). Springer Nature.